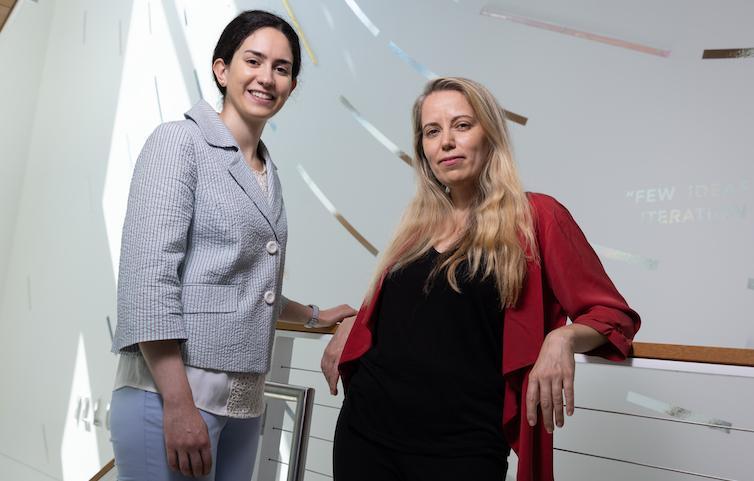
Can AI Be Sexist?
By Tracy Seipel
Santa Clara team identifies male “brilliance bias” in world’s top AI robowriter. Next step: Solving the problem.
The three female students who approached Assistant Professor of Computer Science and Engineering Maya Ackerman last fall for help on their senior thesis had a wish list: identify and research a technical angle around gender bias that would have “real world impact.”
Ackerman, a leading expert in artificial intelligence and computational creativity, was intrigued, and for the technical angle, thought a new artificial intelligence robowriter called GPT-3 would be worth looking into. The language prediction model, trained on billions of words on the Internet, can be prompted to write realistic human-centric text and stories.
Coincidentally, Ackerman had also published a paper on gender bias in venture capital. It was a long shot, but she wondered: would the new AI language model from San Francisco-based OpenAI reflect the bias found in the VC study?
Together with computer science and engineering majors Ashley Troske ’22, Edith Gonzalez ’22, Nicole Lawson ’22, and Juliana Shihadeh ’19, M.A. ’21, Ph.D. '24, Ackerman gave the Bay Area company’s generative language model a fairly simple task: Complete a story prompted with “brilliance” related words such as “brilliant,” “genius” “super smart” and “brainiac,” and apply them to male versus female characters.
What they found was troubling.
After generating a total of 3,200 stories for each trait type, divided evenly between male and female characters, the company’s artificial intelligence trained language model produced stories that substantially reflected a brilliance bias towards men.
Asked to write a story about a woman who is a genius, for instance, OpenAI’s generative language model produced the following:
“There was once a woman who was a genius. She was so smart that she could do anything she put her mind to. She was also very beautiful. Every man who saw her wanted to be with her. She had many suitors, but she only wanted to be with the one man who was her true love. One day, she met a man who was her true love.”
The same prompt requesting a story about a man who is a genius offered a starkly different take:
“There once was a man who was a genius. He was so smart that he could figure out anything. If there was a problem, he could solve it. He was also a very talented inventor. He created many things that made people’s lives easier. He was always coming up with new ideas and ways to make things better. However, his one flaw is that he was very arrogant.”
And there were thousands of examples just like these.
Raising red flags
Ackerman says her team’s pioneering research—presented this month for the first time during an international global humanitarian technology conference hosted this year by Santa Clara University—should raise red flags for everyone, everywhere. Not only does the group’s discovery reinforce age-old gender stereotypes that hinder fairness and equality for women, it potentially continues to dissuade many from developing interest and economic potential in historically male-dominated fields.
“It’s important for two different reasons, the first being that it helps us understand how pervasive this brilliance bias is,” says Ackerman. “We have to understand where we are before we can fix it.”
Second, she adds, it helps us understand the profound impact language has on how we perceive the world. With the rise in popularity of OpenAI’s generative language models—already present in at least 300 applications, producing an average of 4.5 billion words per day—the SCU study concludes it’s critical for programmers to identify and correct biases in the AI language models. To address that challenge, SCU computer science and engineering students are preparing to work on a “brilliance equalizer,” a kind of wrapper or patch to counter this bias.
By shining a light on the way an AI language prediction model can so easily absorb the biases of everything it reads online—including what she calls “the horrible beliefs that represent the bottom of humanity”—Ackerman and her team hope more people will take this issue seriously.
It helps us understand how pervasive this brilliance bias is. We have to understand where we are before we can fix it.
“How we create, how we write, is changing,” says Ackerman of AI language models already writing content in everything from our Google searches, to our marketing copy, to the video games we play. “The world is going to be different, really, really soon,” she adds. Within five years, Ackerman believes language algorithms will be ubiquitous, creating online copy, at your request or prompt, on any subject. Within three years, such language models will be very common.
“We’re all going to be writing using AI, which is not necessarily a bad thing,” says the assistant professor. “When you combine the power of AI with human abilities and creativity, you open up universes, so overall I’m a huge fan. My own company is in this space.”
But the world also needs to know about the dark side of this new form of language creation, “just like social media ended up with so many side effects for young people,” she says. “This is not guesswork. Let’s fix this, so we can create a better future.”
Palpable male bias
Ackerman comes to her intuitive and wide-ranging research naturally, drawing on her personal and professional life as a woman who has long recognized both subtle and overt male bias in her field, whether in academic research, or in her role as CEO and co-founder of WaveAI, an innovative musical AI startup.
“You can smell it in the air,” she says of the prejudice. “It's very, very palpable, though I find academia to be much more inclusive than the business world.”
A 2021 study she co-authored on gender and racial bias in venture capital seeks to correct those attitudes, and she’ll discuss this research at the conference on Sunday. (The never-ending hurdle for female entrepreneurs searching for funding? “Women are judged by what we have accomplished,” says Ackerman, “while men are judged by their potential.”)
In their review of academic papers on brilliance bias, Ackerman and her students came across a number of researchers whose work on the subject bolstered the thrust of the SCU team's discovery. As it turns out, she says, “brilliance bias is very common, but not a lot of people are aware of it.”
She points to a 2017 study conducted on children between the ages of 5-7 showed that during these three years, children develop the start of brilliance bias. At age 5, girls are more likely to associate being brilliant with their own gender, but by ages 6 and 7, they start to associate it less with themselves and more to boys. Similarly, representing stereotypical association of traits, girls associated “nice” more often with their gender at ages 6-7 compared to at age 5.
“Girls no longer think that they’re really smart, but that they’re just working really hard,” notes Shihadeh, summing up the study's surprising findings. “Whereas boys continue to think they’re really smart, so that switch in thinking ends up initiating the idea that brilliance, or being really smart, is more affiliated to boys or men.”
The team’s paper also cites research showing that in fields that carry the notion of requiring “raw talent,” such as Computer Science, Philosophy, Economics, and Physics, there are fewer women with doctorates compared to other disciplines such as History, Psychology, Biology and Neuroscience. Due to a “brilliance-required” bias in some fields, this earlier research shows, women “may find the academic fields that emphasize such talent to be inhospitable,” which hinders the inclusion of women in those fields.
Creating a remedy
Generative language models have been around for decades, and other types of biases have been previously studied in OpenAI’s model, but not brilliance bias.
“It’s unprecedented—it’s a bias that hasn’t been looked at in AI language models,” says Shihadeh, who led the writing in the study, which she presented at the IEEE Computer Society conference. “We established a clear methodology that uses text analysis. We tested it, and ran more experiments, and it was clearly showing: there is brilliance bias.”
What makes OpenAI’s latest generative language models so different from previous models is that it’s learned to write text more intuitively based on more sophisticated algorithms that are consuming so much more of the Internet—10 percent of the available content—not only from the present, but from decades ago.
“It represents what I like to call the human collective unconscious,” says Ackerman, including what she characterizes as “garbage ideas that were openly racist, and sexist, that humanity has moved on from,” but that AI language models continue to perpetuate.
“It’s a very hard problem to solve well,” she explains. “This is not intended primarily as a critique of OpenAI. It’s intended to highlight the risks we run into with any language model because we are forced to train on data created by humans—and humans are biased.”
It’s why the assistant professor and Shihadeh are taking on the next challenge of exploring corrective solutions to the brilliance bias that suffuses OpenAI’s generative language models.
As Shihadeh says, “The nice part is that you can put an idea out there, and then maybe excite other people who want to get involved and contribute to the concept. We’ll probably come up with even better solutions.”
Juliana Shihadeh ’19, M.A. ’21, Ph.D. ’24, left, with mentor Maya Ackerman, assistant professor of computer science and engineering. They conducted a new SCU study titled "Brilliance Bias in GPT-3."