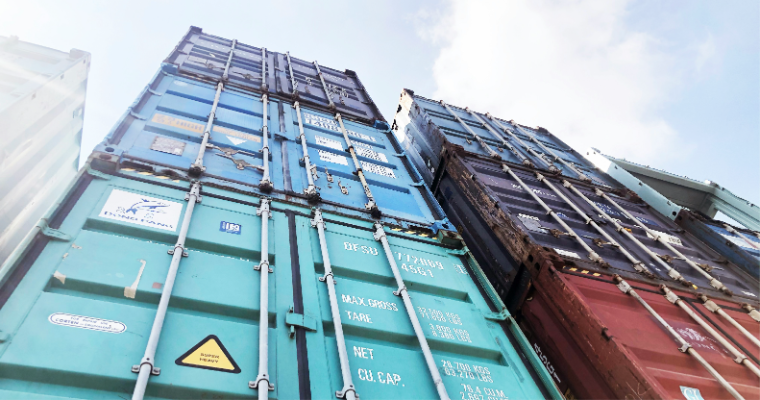
How to Better Utilize Machine Learning in Supply Chain Management - Professor Agrawal Introduces a New Approach
In recent years, the themes of machine learning, artificial intelligence, and supply chain management have been dominating public conversations. Events like the Covid-19 pandemic, the Suez Canal blockage in 2021, and the Russia-Ukraine War have sensitized the general public to the importance of supply chain management and identified a need for improvement that may be addressed by advancements in AI/ML technologies.
“There’s a solid case to be made for focusing on the intersection of these two developments,” explains Naren Agrawal, professor of Information Systems and Analytics, who has conducted extensive research on supply chain issues in the retail and high technology (A&D, semiconductor equipment, telecommunications, and consumer electronics) industries.
“Recent data released by executives at McKinsey suggested that the highest cost saving potential for AI/ML techniques is in the area of supply chain management, and that is really what the focus of my most recent paper is. We help answer the key question - How can companies leverage AI/ML technologies to build supply chains that are agile and resilient.”
Though the use of AI/ML in supply chain management is not new, thus far, machine learning has primarily been used to facilitate visibility in multi-tiered supply chains, improve forecasts, and simulation, or what-if analysis. None of these developments help much with supply chain planning, which is the key component for establishing agility and resilience in supply chains.
Agrawal’s most recent paper, published in the Harvard Business Review, introduces a new approach that is a radical departure from how machine learning is currently used in practice. The optimal machine learning (OML) model is able to replace the conventional, forecast-based, two-step, predict then optimize (PTO) approach with a one-step decision making engine. Bypassing the flawed first step of the PTO system, the OML model connects historical data to decisions that align with the company’s key performance indicators (KPI), going directly from data to decisions.
The second unique component of the OML model is what Agrawal calls the “digital twin”, which is a digital representation of an entire supply chain network, including material, financial, and information flows. Using highly granular, transaction level data in such a digital twin allows companies to rigorously analyze detailed outcomes in a broad range of scenarios, something that is nearly impossible in traditional scenario planning approaches. This capability is critical to assessing and building resilience in supply chains.
“Another major pitfall of the traditional PTO model addressed by the OML model has to do with data,” explains Agrawal. “While our ability to store and access massive amounts of data has sky-rocketed, in practice, this data tends to be highly dispersed across supply chains, and in a format that makes it difficult to use them effectively. Moreover, the traditional ML approach involves throwing as much data as possible at a model and letting it figure out what is important and what isn’t. You get some answers using this approach, but interpreting answers is difficult, and when users don’t understand why an answer is what it is, they don’t feel confident using those answers. By only using relevant and specific data, OML model answers tend to be very interpretable, which helps with improved accuracy of decisions and user acceptance.”
Agrawal and his co-authors put their approach to the test and implemented their model in two Fortune 500 companies. The first was at a semiconductor equipment manufacturer that, after running the model, found they were able to increase their service level by over 5% without any significant increase in inventory or operate at the same level of service with significantly less inventory.
An OEM company that manufactures consumer electronic goods also implemented the OML model to identify key opportunities for improvement in their inventory strategy. The methodology identified significant imbalances between demand and current inventory policies. For example, the company would routinely have shortages even though they had the inventory, but it was always at the wrong location. By using the OML methodology, they were able to quickly recognize these imbalances and recommend planning decisions that could help address these shortages.
Agrawal hopes that other companies can leverage this new approach, as across all industries, creating agile and resilient supply chains is ultimately the need of the day. This is what will allow companies to reduce their costs and increase revenues, profits and customer satisfaction efficiently.
“We have made a ton of progress over the last 30-40 years in how we think about managing supply chains, but there is still room for further improvement, " says Agrawal. “Many of the shortcomings of the traditional methods have to do with how technology and science are applied, which is what we aim to address in the OML approach. It required us to step back and think differently about the problem, and that is what makes our new approach distinct.”
Read Professor Agrawal’s full research: How Machine Learning Will Transform Supply Chain Management.