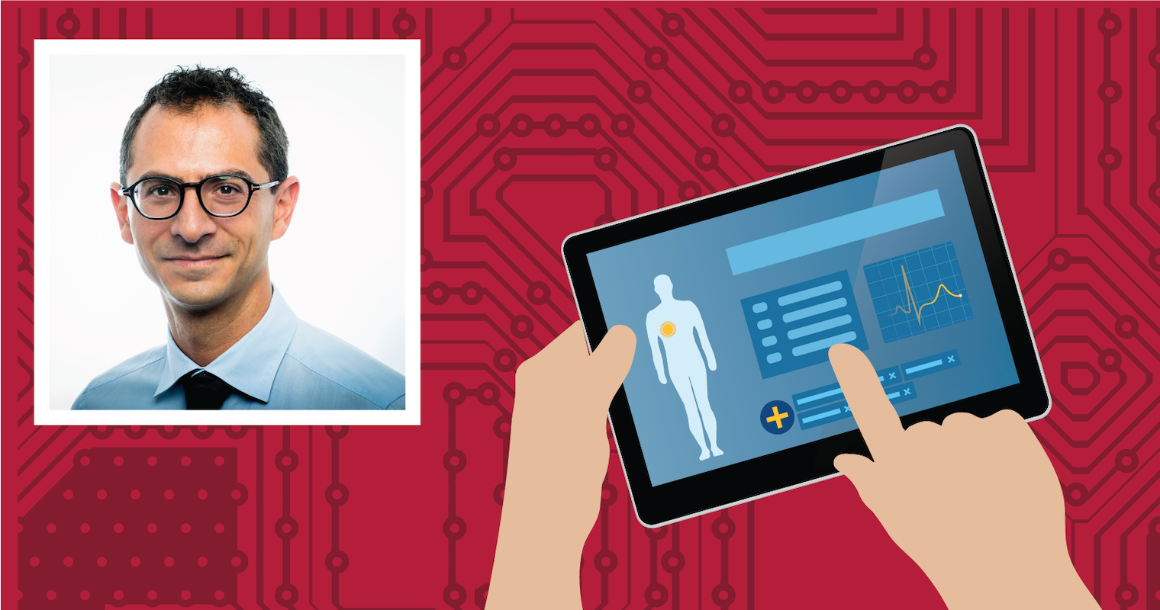
Leavey Associate Professor Michele Samorani Leads the Charge to Uncover Bias in the Use of Artificial Intelligence
While generative artificial intelligence (AI) has been causing a recent buzz with the introduction of programs like ChatGPT, the use of AI is not an entirely new concept. For years, the healthcare industry has been leveraging AI and machine learning (ML) to diagnose, treat, and monitor patients, make more accurate predictions, optimize scheduling, and increase efficiency.
Leavey School of Business Associate Professor of Information Systems and Analytics, Michele Samorani, has been examining the use of machine learning in medical appointment scheduling for years. Back in 2019, Samorani was presenting at the National Academies of Sciences, Engineering, and Medicine when a practitioner brought to his attention an unsettling trend in his research. While machine learning and no-show probability can be used to optimally schedule medical appointments, it is correlated with the patient’s socioeconomic status.
“One major lesson I learned and a takeaway that I encourage people to keep in mind, especially with the increasing use of AI in business and society, is that there is always a risk of bias when using AI/ML to make predictions about people, whether it is medical appointments, credit card approvals, or the movies and TV a platform advertises,” said Samorani.
Since then, he has been researching bias in AI and has brought on board Leavey professors Haibing Lu (Information Systems & Analytics) and Michael Santoro (Management, Business Ethics), as well as Shannon Harris (Virginia Commonwealth University) and Linda Goler Blount (Black Women’s Health Imperative). The full team has embarked on a new study to develop appointment scheduling methodologies that minimize racial disparity while maximizing clinic efficiency - a study years ahead of federal policy on bias in AI.
The award-winning research, “Overbooked and Overlooked: Machine Learning and Racial Bias in Medical Appointment Scheduling,” found that state-of-the-art appointment scheduling systems generally use predicted no-show probability risk strategies to assign patients to appointment slots, meaning a patient who is statistically expected to have a higher risk of being a no-show is assigned a less desirable time slot.
“However, we know that those who have a higher probability of not showing up to their appointments also typically have a lower income, can’t afford childcare, are single parents, have multiple jobs, or don’t have reliable transportation to their appointments,” Samorani noted. The study, which focused on Black and Nonblack patients, found that Black patients waited 30% longer than Nonblack patients, which further increased negative feelings towards healthcare among a population that is already historically underserved.
In fact, there are multiple types of bias risks in AI/ML that can arise from a wide range of causes, including from the people who develop the system, to the prediction auditing process, to how individuals are using these predictions in real situations. Algorithms used in the operations management field are very popular, have been used for years, and have a common, well known objective function: maximizing efficiency. However, as Samorani’s research demonstrates, maximizing efficiency can often exacerbate pre-existing biases.
“It is important to note that bias in AI is not a new concept,” said Samorani. "There was actually an instance at Amazon about 10 years ago when the company was forced to stop using an AI recruiting tool that displayed bias against women.”
Now that these technologies are becoming more widely available and utilized, Samorani believes it has never been more important to implement laws and regulations that ensure systems are identifying and combating bias. He explained, “Businesses have long strived for workplace equality, and it is imperative that emerging technologies do not diminish these accomplishments. In raising awareness about the need for regulation, we can get ahead of major implications biased systems could have on society.”
As for the future of AI/ML, Samorani said, “I hope that researchers and platform creators will revisit existing algorithms and develop ways to prevent or detect bias ensuring there is equal representation across new systems.” At Leavey, Samorani and his colleagues remain focused on uncovering bias and have already begun a follow up work that further studies the unintended disparities that arise from employing AI/ML software to optimally schedule medical appointments.