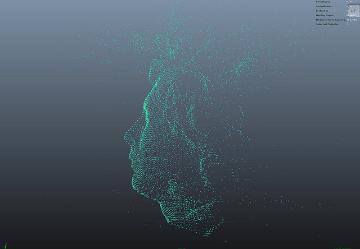
Employee/applicant data: the interplay between privacy and diversity efforts
On February 27, 2018, Privacy Lab (which is “a meetup for privacy-minded people to foster communication and collaboration”) was co-hosted by the Markkula Center for Applied Ethics and the High Tech Law Institute, at Santa Clara University. The description of the event explained its focus:
With underrepresentation of diverse groups in the tech industry, and given that diverse workplaces can lead to better products and more successful companies, many Silicon Valley companies are undertaking efforts to improve diversity in their workforce. However, this requires the collection of information (from both applicants and employees) that can be considered sensitive, such as ethnic origin.
In the U.S. many companies believe that ensuring diversity justifies information collection. However, the laws or norms in other countries may not allow or severely restrict collection and use of such information. How do companies address this potential conflict? In addition, if they can collect such information, how do they protect it, especially given that some data sets are so small that they can’t really be anonymized for reporting purposes? And how do they give people control over information about themselves, including the right to revoke or delete it? Join us for an important conversation about the tension between two competing goods.
The panelists were Alicia Gray and Shoshana Isaac (from Mozilla), and Jackie Wilkosz and Susana Fernandez (from Intuit). Colleen Chien, associate professor at Santa Clara's School of Law, moderated the panel. The views expressed were not necessarily the views of the companies or the particular speakers, and the discussion was held under Chatham House rules. Below are the summary notes from a conversation that offered, according to audience reviews, “great practical tips and overviews,” and “fantastic and insightful information.”
Summary notes
The panelists started by clarifying a few underlying assumptions: that diversity is good, and that, when implemented in companies, it leads to better business outcomes.
In the US, the EEOC and various laws impose affirmative requirements for the collection of certain diversity data—e.g. about sex and race (or “ethnic categories”). But the terminology is not consistent across such laws; for example, some mention “sex,” others “gender” (but not both). If an applicant doesn’t provide the data, the potential employer is required to visually identify characteristics like “ethnic category” and fill in the data. And the categories are often restrictive and binary.
One issue that most laws don’t address is the transition from applicant to employee; what happens to the data of the same person as he/she moves from one category to another?
(A question related to this was how long you should/can retain applicant data after the hiring decision is made. The answer depends on the jurisdiction. Six months, for example, might be too long in a European country but too short in the U.S.)
Tech companies typically capture diversity data during the application and on-boarding processes, and tend to classify such data as highly sensitive. Some companies offer an option not to disclose. Some companies maintain data using service provided by 3rd party vendors (e.g. Workday). In some, employees retain the ability to directly edit such data. Some companies run annual campaigns to update the databases.
There are three use cases for data collected as part of D&I efforts: 1) affirmatively use that data in order to drive diversity; 2) be conscious of that data but use it only as a tie-breaker (though question flagged--how does one assess different candidates with different strengths, backgrounds, experiences, so as to reach a “tie”?); 3) simply to create consciousness informed by data. For 1 and 2, that means companies undertaking such uses have accepted the risk that they might be sued for discrimination, since there are legal restrictions on making hiring/promotion decisions based on protected categories.
Another related question: if your applicant pool is diverse but your hiring decisions don’t reflect that diversity, do you have a bias issue you need to address? How?
It’s important to have transparency re. what you use D&I data for. What does D&I mean in your organization? Otherwise people will make assumptions and worry about your uses, and will likely try to share as little as possible. Your D&I program is no good if people won’t participate in it—so you need trust.
Under GDPR, the European law that’s about to come into effect in May, the types of data used for D&I programs are deemed to be “special categories” and require a special legal basis for their processing. You might also be allowed to collect the data if you get meaningful consent from the data subjects, or if you show that the data is necessary for you to carry out obligations under a member state’s law. (So there might be benefits to having clear laws around D&I that require you to collect and use certain data. We might see new member state laws re. diversity that would allow for D&I processing under GDPR.) Consent is rarely deemed valid when given by employee to employer.
Big question: how do you institute a global D&I program, given that different jurisdictions demand or prohibit the collection or use of different kinds of data? In France, for example, there is an outright prohibition of the collection of race data--but see EEOC requirement in the US, mentioned above. The French prohibition is based on the underlying focus on equality. But while stressing that value, the law makes certain D&I efforts (aimed at promoting equality) impossible.
Risks: In the US, if you are an entity under obligation to report data and you don’t do it, you will get hit with fines. In the EU, the threatened penalties under GDPR are very high. Additional risks come from indirect collection: you need to consider what other info your organization is collecting (e.g. HR recruiters may be scraping LinkedIn, and the association of individuals with specific LinkedIn groups may de-facto disclose ethnicity or other protected categories).
Some companies try to get around restrictions on collecting certain information by making inferences: for example, they don’t collect gender data but use services that promise to algorithmically determine sex based on applicant names. (Consider the potential of incorrect assumptions and results.)
Suggestions: Do a privacy impact assessment for D&I data, even if it’s not required by law. (GDPR does require privacy impact assessments for “high risk data” and “high risk processing.”)
For risks that can’t be mitigated, even after the data analytics teams work with the privacy legal teams, who do you go to? Do you take this to the C-suite? Identify ahead of time who has the responsibility to ultimately make that decision. In some jurisdictions, companies are required to reach out to regulators in order to make that determination.
Re. risk: Keep in mind that, within businesses, data sets often end up shared among groups. Beware of “secondary purposes” of use of databases. People do want to be mindful and thoughtful about these programs, but they might still seek permission to see data or process it in ways that would be problematic.
The questions underlying both the privacy and employment law concerns are really broad, philosophical questions—about what we value, how we balance competing goods, how we best protect the rights of the individuals involved. As one speaker noted, “you lose good data by implementing respect.”
Suggested best practices:
- Implement Privacy by Design: Consider how to protect before you collect.
- If possible anonymize the data—but consider how that can affect the rights that individuals may have to access and correct their data.
- Avoid secondary purposes: Don’t use this data for anything else.
- Minimize collection.
- Work to ensure that categories allow for accurate identification.
- Impose access controls.
- Ensure that your company has a clear internal policy and procedures that support the policy (Transparency is key; clear, disclosed policies will promote the trust of your applicants and employees.)
- Put in place a Privacy Impact assessment (GDPR requires it because D&I data is high risk).
- Periodically review your need for predefined reports using this data (and get rid of reports that are no longer needed).
- Decide how long the data will be retained, if it’s not anonymized (and be careful with sample sizes, as anonymization will not be possible if the sample is small).
- Segregate such information in order to limit the impact of a potential incident response.
- When managing diversity data, use common sense: Just because you can measure it does not mean that you should.
- Limit sharing of data internally by imposing protective requirements (e.g. minimum requirement of 5 records before running a report; releasing only location to incident response teams responsible for ensuring the safety of employees in a disaster situation; not releasing information to employees who are simply wishing to promote internal groups or networks).
Last but not least, consider the aspects that impact diversity efforts but are either harder to measure or not reflected in the diversity data currently being collected for D&I purposes: the ways in which people of different genders are acculturated, for example; unconscious bias; etc. Inequalities arise much earlier than the start of the “pipeline problem.”
Illustration by Daniel V, used without modification under a Creative Commons license.