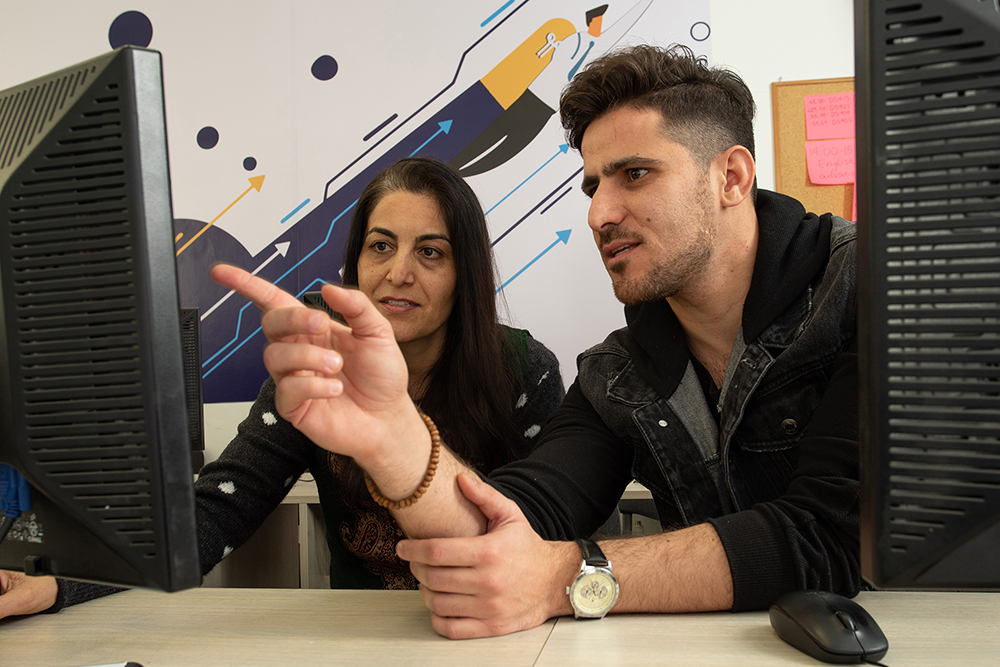
Can Biased Humans Design Biased Algorithms that Still Enhance Human Flourishing?
Irina Raicu is the director of the Internet Ethics program (@IEthics) at the Markkula Center for Applied Ethics. Views are her own.
The following is a lightly edited version of comments made as part of a panel discussion at Duquesne University in October 2022. The panel was titled “Can Biased Humans Design Unbiased Algorithms?”
Let me start by acknowledging that “bias” is a loaded term. We could have a whole conversation about how the term has a different meaning in statistics than it does in general discussions, about negative connotations, definitions, and the difficulty of talking across disciplines. But that’s not the conversation I think we want to have. I would like to propose that we talk not about bias but about “subjectivity” instead: about all the subjectivity that’s embedded in AI systems that are often, wrongly, portrayed as “objective.”
So, I might rephrase the title as “Can we eliminate subjectivity from algorithms, or, more broadly, from AI systems?” And then it becomes easier for me to argue that the answer is “no.”
Think of this as a kind of annotated reading list, for those who want to learn more about each of the points that I’m just going to sketch quickly. I have ten quick points to make, and will flag nine readings.
1. Datasets and algorithms are both human artifacts. They reflect human choices: choices made in terms of what data to collect, how to categorize it, how to “clean” it, etc.—and choices made in the design of the algorithms themselves.
They embed subjective decisions, in other words, and they measure and assess only subsets of reality. The boundaries of those subsets are determined by human beings.
But they also reflect human choices made by the broader societies within which the data is collected. Data about arrests, for example, reflects many societal choices about policing, and choices about what drugs to criminalize or decriminalize. Data about employment reflects societal choices about which people fit into which roles.
2. There are other points where subjectivity enters: for example, when we decide which issues we want to address with algorithmic tools in the first place. A few years ago, to make this point, technologists working for a magazine called New Inquiry came up with an algorithm to identify white collar crime risk zones. This, of course, challenges implicitly the choices made in current deployments of predictive policing, which target particular “traditional” crimes.
The authors released their tool and a related white paper, in which they added,
Recently researchers have demonstrated the effectiveness of applying machine learning techniques to facial features to quantify the ‘criminality’ of an individual. We therefore plan to augment our model with facial analysis and psychometrics to identify potential financial crime at the individual level. As a proof of concept, we have downloaded the pictures of 7000 corporate executives whose LinkedIn profiles suggest they work for financial organizations, and then averaged their faces to produce generalized white collar criminal subjects unique to each high risk zone. Future efforts will allow us to predict white collar criminality through real-time facial analysis.
Thinking along similar lines—if we use AI in hiring, why are companies not using AI in the process of appointing CEOs? (To be clear, that is a question, not a recommendation.)
3. That mention of the 7,000 pictures of corporate executives brings us back to datasets. On this, I would recommend an essay by Deborah Raji, which appeared in the MIT Tech Review, called “How Our Data Encodes Systematic Racism.”
Raji writes, “I’ve often been told, ‘The data does not lie.’ However, that has never been my experience.” She then asks,
Tell me—what is the difference between overpolicing in minority neighborhoods and the bias of the algorithm that sent officers there? What is the difference between a segregated school system and a discriminatory grading algorithm? Between a doctor who doesn’t listen and an algorithm that denies you a hospital bed?
In her article, all of those references to different algorithms are links—links to other articles about algorithms that have already been deployed in our communities. Algorithms that are already playing a role in shaping our current reality, in turn influencing the data that we might collect then for future work.
Although Raji’s questions are rhetorical, critics of current AI deployment would say that one difference between discriminatory practices and discriminatory algorithms is the obfuscation of discrimination behind veils of math. They would refer to this as “bias laundering.”
In terms of articles about algorithmic bias deployed at scale, we have many such examples by now. One of the people whose work has been seminal in bringing awareness to algorithmic harms is Cathy O’Neill, the author of a book called Weapons of Math Destruction, published in 2016. It is very accessible, and I highly recommend it for anyone interested in questions about biased algorithms. I recommend it in part because it will make you angry—angry that we’ve been warned since 2016 and we still allow systems to be deployed, usually in the name of efficiency but also with a purported interest in objectivity, even where they cause harm at scale.
4. Data categorization and labeling are other points where subjectivity enters. One striking example is detailed in Kate Crawford’s book Atlas of AI. In it, she discusses ImageNet—a dataset that’s been the foundation of many object or image recognition algorithms. As Crawford explains, ImageNet initially (and for a decade) contained more than 2,800 subcategories under the category of “Person.” Among those subcategories were “debtor,” “boss,” “color-blind person,” but also, “failure,” “hypocrite,” “slut,” and “unskilled person.” These were labels for images of people.
After 10 years, in 2019, the ImageNet Roulette project led by artist Trevor Paglen drew attention to this. The response was an effort that led to the elimination of 1,593 subcategories.
However, Crawford points out that ImageNet still contains (or did at the time of her book’s publication), distinct subcategories for “assistant professor” and “associate professor” (as if, she points out, a promotion might lead to biometric changes in one’s face).
“Classifications,” Crawford writes, are “unavoidably value laden” and “force a way of seeing onto the world while claiming scientific neutrality.”
5. By now most researchers and many policy makers are aware of issues of biased datasets, but, again, that’s not the full scope of the problem. There’s a great Twitter thread by researcher Sara Hooker, in which she unpacks why algorithmic bias is not purely a data problem. As she puts it, “choices around model architecture, hyper-parameters, and objective functions all inform considerations of algorithmic bias.” The thread links to research papers, for those who again want to delve deeper. And I’m not a technologist, so I’m not in the best position to explain all of that.
By the way, panels that address AI ethics should always include a technologist. Technologists need to become public speakers. We need the realism of technologists to counter the hype pitched by other technologists. Without their input, we can’t get our societal responses right.
6. What about efforts to debias algorithms? I think they are important, but their limitations need to be understood and clarified, just like the limitations of AI in general need to be.
For example, one of the areas in which algorithms have been and are being deployed with deeply problematic effects is the criminal justice system. But we can think of the whole criminal justice system as an algorithm. For a very long time now, people have worked to adjust its parameters and procedures in an effort to “unbias” it. Those efforts are important, and are ongoing, but they have not succeeded in eliminating subjectivity from the system.
7. On a related topic, by the way, I would recommend the “Report on Algorithmic Risk Assessment Tools in the U.S. Criminal Justice System,” published by the Partnership on AI in 2019.
Here’s a bit from that:
Decisions regarding what data to use, how to handle missing data, what objectives to optimize, and what thresholds to set all have significant implications on the accuracy, validity, and bias of these tools, and ultimately on the lives and liberty of the individuals they assess.
In addition to technical concerns, there are human-computer interface issues to consider with the implementation of such tools. Human-computer interface in this case refers to how humans collect and feed information into the tools and how humans interpret and evaluate the information that the tools generate. These tools must be held to high standards of interpretability and explainability to ensure that users (including judges, lawyers, and clerks, among others) can understand how the tools’ predictions are reached and make reasonable decisions based on these predictions.
This draws our attention to the fact that we can’t address subjectivity only in the context of algorithm design—we need to address it in algorithm deployment, too.
8. That brings us to the human-in-the-loop issue. If we can’t design objective algorithms, some propose to address this either by placing humans in a fail-safe role of overriding specific bad algorithmic decisions or suggestions, or by keeping humans in the role of ultimate decision-makers, with the algorithms just there to offer an assist.
This is where we might talk about studies showing that humans are reluctant to override decisions made by algorithms. See, also, articles exploring the limits of the human-in-the-loop approach.
But what I’d like to stress is that I find myself increasingly troubled by the term “human in the loop”—referring, as it does, to this kind of final arbiter role. When it comes to algorithms, as we’ve just seen, it’s humans all the way down. There are humans in the loop from the very inception, the very conception of the loops.
9. Given that, it is deeply important to bring even more people into the “loop” by helping those who are not data scientists or machine learning experts to understand more about those loops. Just this week, for example, I came across a great essay titled “How to Read an AI Image: The Datafication of a Kiss,” by artist and AI researcher Eryk Salvaggio. It is an analysis of an AI-generated image of two people kissing. Salvaggio begins by explaining that “AI images are data patterns inscribed into pictures, and they tell us stories about that dataset and the human decisions behind it.” He carefully explains what he means by that, in a way that, again, like almost all of the other writing mentioned here, is accessible to those of us without a technical background. He then adds that careful analysis of those patterns inscribed in pictures “moves us ever further from the illusions of ‘neutral’ and ‘unbiased’ technologies which are still shockingly prevalent…. That’s pure mystification. They are bias engines. Every image should be read as a map of those biases, and they are made more legible through the use of this approach.”
We all need to learn how to read the bias maps.
10. So, can biased humans design unbiased algorithms? In anticipation of this panel, I had a conversation with some of my colleagues at the Markkula Center. I told them that I wanted to answer “no.” At least one of the engineers thought that would be a presumptuous answer (though he put it in a nicer way). But maybe there’s presumptuousness baked into the question itself.
In our conversation, my colleagues pointed out that we can use data and algorithms to detect bias. And that we can put in guardrails, and keep adjusting them, to address bias. So maybe a better question would be “Can Biased Humans Design Biased Algorithms that Still Enhance Human Flourishing?”
There is a wonderful free online textbook by Solon Barocas, Moritz Hardt, and Arvind Narayanan, titled Fairness and Machine Learning: Limitations and Opportunities. Its introduction is one of my favorite readings about this topic. Toward the end of the introduction, after detailing many, many issues to be addressed, the authors offer their reason for optimism: they argue
that the turn to automated decision-making and machine learning offers an opportunity to reconnect with the moral foundations of fairness. Algorithms force us to be explicit about what we want to achieve with decision-making. And it’s far more difficult to paper over our poorly specified or true intentions when we have to state these objectives formally. In this way, machine learning has the potential to help us debate the fairness of different policies and decision-making procedures more effectively.
It has the potential, as long as we use it wisely, and without naïve notions about its purported objectivity.
Photo: Nacho Kamenov & Humans in the Loop / Better Images of AI / Data annotators discussing the correct labeling of a dataset / CC-BY 4.0