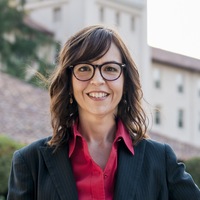
"[G]ood data scientists... never forget the people behind the data and the moral respect that they are owed."
Welcome to "Cookies, Coffee, and Data Ethics"! My name is Jeff Kampfe and I am a senior at Santa Clara University, studying Economics and Philosophy. I am also a Hackworth Fellow at the Markkula Center for Applied Ethics. This article is the second in a series of interviews that involve hot coffee, tasty baked goods, and the complex issue of data ethics. The goal of these interviews is to see what individuals with unique and insightful viewpoints can teach us about the field data ethics, where it is heading, and what challenges we may face along the way. Thanks for stopping by!
The following is an edited transcript of a conversation with Professor Shannon Vallor.
Shannon Vallor is the Regis and Dianne McKenna Professor in the Department of Philosophy at Santa Clara University and an AI Ethicist/Visiting Researcher at Google. Her research areas of expertise are the ethics and philosophy of technology, philosophy of science, and phenomenology. Her current research project focuses on the impact of emerging technologies, particularly those involving artificial intelligence and robotics, on the moral and intellectual habits, skills, and virtues of human beings. She also serves on the executive leadership team of the non-profit Foundation for Responsible Robotics and is a past President of the Society for Philosophy and Technology. Professor Vallor has a special interest in the integration of ethics with industry and in engineering/computer science education, and engages in public outreach on this subject with a range of stakeholders inside and outside academia, including government, industry, law, media, and public policy professionals and advocates.
Can you tell me a bit about your past experience with data science?
My field is actually technology ethics, so I'm a philosopher and ethicist of emerging technologies. My area of expertise has included AI ethics and data ethics for the last 5 to 10 years, following the growth in the field and the emergence of deep machine learning. While I'm not a machine learning researcher myself, whenever there's a new technological advance that carries significant ethical implications, it's something that I'm tracking and writing about. Machine learning is what generated a lot of the recent progress in AI research, which until the last ten years or so had been progressing more slowly. It is the form of AI that is being rapidly integrated into data practice and society.
Is there any particular aspect that makes data analytics and data science different from other technologies?
I certainly think that the aspect of data science that focuses on predictive insights raises some special questions. The ability to act on inferences about the future that are produced by a system that doesn't understand any of the data that it's working with, that doesn't understand the world the data represents, and that doesn't have any relationship of care of with the persons who generated the data is very sensitive. We're transitioning to a place where the kinds of data insights that previously would be drawn by people, people who would have knowledge of what the data represented and have the social context to understand the meaning of the data, are now being made by machines. So there is a real challenge in figuring out to what extent and under what conditions we rely upon projections about events, state of affairs, and behaviors that the machines making the projections don't themselves understand.
What are some of the virtues or values that make a “good” data scientist? What do those look like in practice?
The first would be the virtue of humility. Making sure that as a data scientist, you're not simply focused on what you can predict, what you can control, what you can measure, and what you can analyze. You need to know what you can’t measure, what you can’t control. There must be an understanding of the limits of the tools you're working with and understanding of the ways in which tools do not always deliver the insights that we hope they will. A good data scientist must understand the ways in which the instruments and the algorithms can behave unpredictably, the way in which they may have effects that we didn't intend, and simply the humility to understand that we as researchers are not gods. We will make mistakes and some of those mistakes have the potential to do harm. Our power has to be used responsibly and with restraint.
A second important notion is the ability to understand [that] data is about people. Data points are not about abstract subjects that can be treated as cells on a spreadsheet to be manipulated. Data represents observations and indications of human life and activity. It reveals things about individuals who have moral status, dignity, and the right to be treated as such. I think good data scientists never forget that. They never forget the people behind the data and the moral respect that they are owed.
Can you explain more about how describing people as data points can impact their dignity?
I think we see this all the time when we ignore the outliers and treat the data points that fall outside the curve as insignificant. Those are not always noise or error; they often represent human experiences and stories that may be just as meaningful and may have just as much to tell us as the people who represent the statistical norm. Understanding the people behind the data means understanding that you cannot simply erase the data that are inconvenient. You can’t detach what those data represent from human stories and human experiences that are unique and particular. The labels that we attach to the data are always going to be cruder and less representative of what they describe than what we would like them to be. Treating candidates under a single label, whether it's a gender label, whether it's an age group, whether it's consumers of a particular product, or whether it’s people suffering from a particular disease, can cause people to be treated as interchangeable and fungible datapoints. Every one of those individuals with that label is unique and has the right to be respected as a person.
What are your thoughts on data ownership? What are some of the largest issues that need to be resolved surrounding this topic?
The fact that our data is being collected in a public realm, being collected in a context where we've chosen to share it with certain other people, or being collected in a way that conforms to a terms of use agreement, does not necessarily mean that we ought to be seen as relinquishing ownership of our data. Ownership of data has to do with the ways in which data are extensions of our lives and experiences. These data wouldn't exist in the world without our activity and efforts. These points we generate can reveal parts of ourselves that we have a right not to reveal in ways that we don't control or choose. So part of data ownership is about control over our person, control over the story that is told about us, and control over what can be known about us. The meaning of the data is something that the person who generates the data can never really be detached from fully. To the extent that the data represents aspects of ourselves that we can't be separated from, and to the extent that the data can be used to benefit us, hurt us, expose us, or protect us, then we have to have an ability to understand the stakes of transferring that data. There have to be real conditions, meaningful conditions, placed on its use. It's hard to have those conditions if there's a presumption that we don't own our data or if there’s a presumption that the minute we generate data in a visible space it becomes separable from us as someone else's property.
Is it ethical for organizations (incl. companies, governments, nonprofits, ext.) to keep data sets indefinitely? If not, how should that issue be addressed?
I think [keeping datasets indefinitely] is a habit of convenience that carries significant risks and, in many cases, can't be justified. It's worth noting that the Association of Computing Machinery, the largest organization of computing professionals, updated their code of ethics this summer for the first time in decades. One of the changes that they made was to include in the code of ethics a commitment to a minimalist approach to data collection and storage. The default practice of “collect it all, store it all, for what purpose we do not know, but let's have it on hand so that we can use it later should we find a purpose” has been explicitly rejected by the ACM code of ethics. I think that's an indication that this is an old habit of data practice that was probably never justified. We know the risks well enough now to know that it's irresponsible practice.
So how do those risks manifest themselves?
An obvious way is privacy risks. If you have large datasets about lots of people that can potentially be used to identify individuals, or even if they are anonymized, the fact that they can be combined with other datasets and be de-anonymized means that there's no way to safely store data about individuals. There is always a potential for data exposure. You wouldn't store something inherently risky like fuel or an explosive indefinitely; you wouldn't store it without having good reasons for having it on a site. The same is true with data. It ought to be treated as something having inherent risk attached to it, and having data in your possession requires that you be able to defend not only its collection, but the way in which it is stored and how long it’s kept.
How might we as a society balance the benefits that data analytics gives us (in health care, security, efficiency, innovation) against some of the potential threats it poses to privacy and autonomy?
Well I think the answer to that comes from looking at who is the “we.” The “we” that benefit from data practices aren’t always the same people as the “we” that are at risk from data collection practices. So when you say “Can we justify the risks by appealing to the benefits we receive,” that sounds like it's the same people. Yet it’s often not. It’s often the people who are at risk who aren't benefiting at all from the data, and it's often the case that they are the ones with no say over what happens with their data. So I think part of what we need is a bottom-up process in which the people who are at risk from the data have an appropriate voice in the conversation and arguably should have the loudest voice. Whereas the people who benefit from the data ought to be able to benefit only with the consent of those who are at risk. Presently that's not the way the system is designed.
What methods could be used to use to give people who are being affected by data science a louder voice?
I do think that one avenue is regulation. If you have regulators who are responsive to citizens, who are responsive to all the stakeholders, who are well-informed about the technology, and who can act in the public interest, then regulators can seek input from those stakeholders. They can work with advocacy groups including privacy advocates, but also groups of particular individuals who tend to be disproportionately impacted by irresponsible data practice. Those individuals can have a voice in the regulatory process, but you have to be able to trust that you don't have a situation of regulatory capture. Basically, when the regulators are representing and listening only to paid lobbyists rather than the people who actually are the most directly affected stakeholders, there seems to be a problem. The political process is supposed to work in such a way that people can have a voice by giving input.
We can also put pressure on corporations. There's a lot of grassroots efforts from groups and individuals who feel that they are being disparately impacted and put at risk by these technologies without compensating benefits. Those individuals can put pressure on companies to do better or put pressure on academic researchers and data scientists to be more responsible with their practices. Public criticism of research or products released that clearly represent careless or unethical data practice can be quite effective.
Certainly education is important as well, so that affected communities can better understand their rights and better understand the tradeoffs involved with participating in the data economy. But increasingly a lot of us are participating in the data economy without being asked, so increasingly we’re living in a digital surveillance state where information is being gathered about us in ways that we don't explicitly consent to. So that's where the political process needs to function to limit those forms of overreach.
For a prior article is this series, see "On Data Ethics: An Interview with Jacob Metcalf."