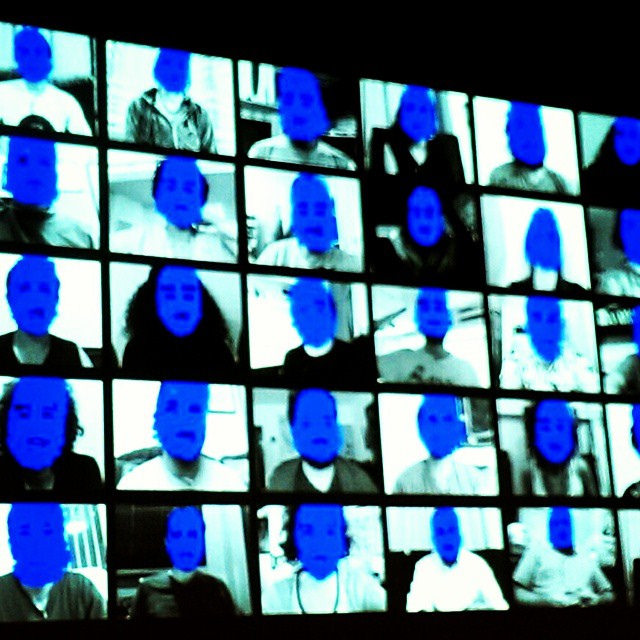
An Educational Experiment
Irina Raicu is the director of the Internet Ethics program at the Markkula Center for Applied Ethics at Santa Clara University. Views are her own.
Over in Santa Clara University’s Leavey School of Business, professor Sanjiv Das teaches machine learning to graduate students enrolled in the MS of Information Systems program. As the Spring 2017 quarter was about to start, Subramaniam (Subbu) Vincent (the Tech Lead for the center’s Trust Project, and an engineer-journalist with experience in data science) suggested that the two of them might collaborate in an effort to introduce the students to some key questions in data analytics: what do fairness and bias look like in the context of machine learning? And, if bias is detected in a dataset or an algorithm, are there ways to minimize or correct for it?
In his hands-on, skill-building course, professor Das asked the students to work in small groups as they practiced predictive modeling on data sets—and proposed the fairness questions as one project option. Five of the groups took him up on the offer. They worked with several databases that included criminal justice and loan data (some of those sets were public and available at no cost; others were licensed for educational purposes only). Subbu and Sanjiv also introduced the students to Themis-ml: a "’fairness-aware’ machine learning interface,” as its creator Niels Bantilan describes it, “which is intended for use by individual data scientists and engineers, academic research teams, or larger product teams who use machine learning in production systems.”
At the end of the quarter, all of the groups presented their findings to the class as a whole. More than 80 students, including those who had not chosen projects focused on the fairness questions, got to hear the results of the application of Themis-ml—and other classification models—to a variety of data sets. What they heard, from their peers rather than from either business professors or ethicists, was that the data showed, indeed, evidence of bias. And that particular machine learning interfaces had various levels of success in countering that bias.
The word “ethics” came up in only one of the presentations, and even “fairness” took a while to make an appearance (though “discrimination” did). Interestingly, the term “consistency” seemed to serve as a proxy for “fairness." In some cases, the students presenting their results noted that an improvement on the consistency axis had come at a modest cost to the accuracy of the prediction. In others, though, the effort to remove bias had actually improved the accuracy of the model.
(Maybe that result should have been expected: if bias is defined as “prejudice in favor or against one thing, person, or group, usually in a way considered unfair,” and prejudice is defined as “a preconceived opinion that is not based on reason or actual experience,” it makes sense that removing prejudice would lead to more accurate predictions—about, say, which people convicted of a crime might actually re-offend, or which borrowers might default on their loans.)
I and my colleague Brian Green (the director of the Technology Ethics program here at the Center) attended the presentations, and tried to gauge the student’s reactions. As they heard that bias might lead to women or Hispanic Americans being less likely to get small loans than white men would, or to African Americans convicted of a crime being deemed, inaccurately, more likely to re-offend than white defendants similarly situated, and that there were ways of limiting the impact of bias when working with datasets that reflected societal biases, even the students who hadn’t chosen projects with an overt “ethics angle” paid close attention.
In professor Das’ class, several dozen students got a hands-on, skill-based lesson in applied ethics—without, perhaps, realizing that they had. They learned that databases are not an accurate reflection of the world as it is, but subsets of information that may well encode biases. That “data-based” doesn’t mean “objective.” That there are data scientists actively working to address this problem. And that, armed with such awareness, data science professionals can actually combat unfairness, rather than ignore it, perpetuate it, and/or cover it up under a layer of “math.”
It was an educational experiment. If you are teaching data science/machine learning courses and decide to recreate it in your courses, or if you have tried your own experiments in introducing questions of ethics into such courses, we would love to hear about your experiences. Given the growing application of machine learning to all manner of decisions about our personal lives and our societies, we need new ways to ensure that machine learning expertise comes with awareness of the ethical implications of such work.
For more details about this experiment, see "How Might Data Science Students Consider Ethics?" by Subramaniam Vincent and Brian Green.
Photo by Sheila Scarborough, used, without modification, under a Creative Commons license.