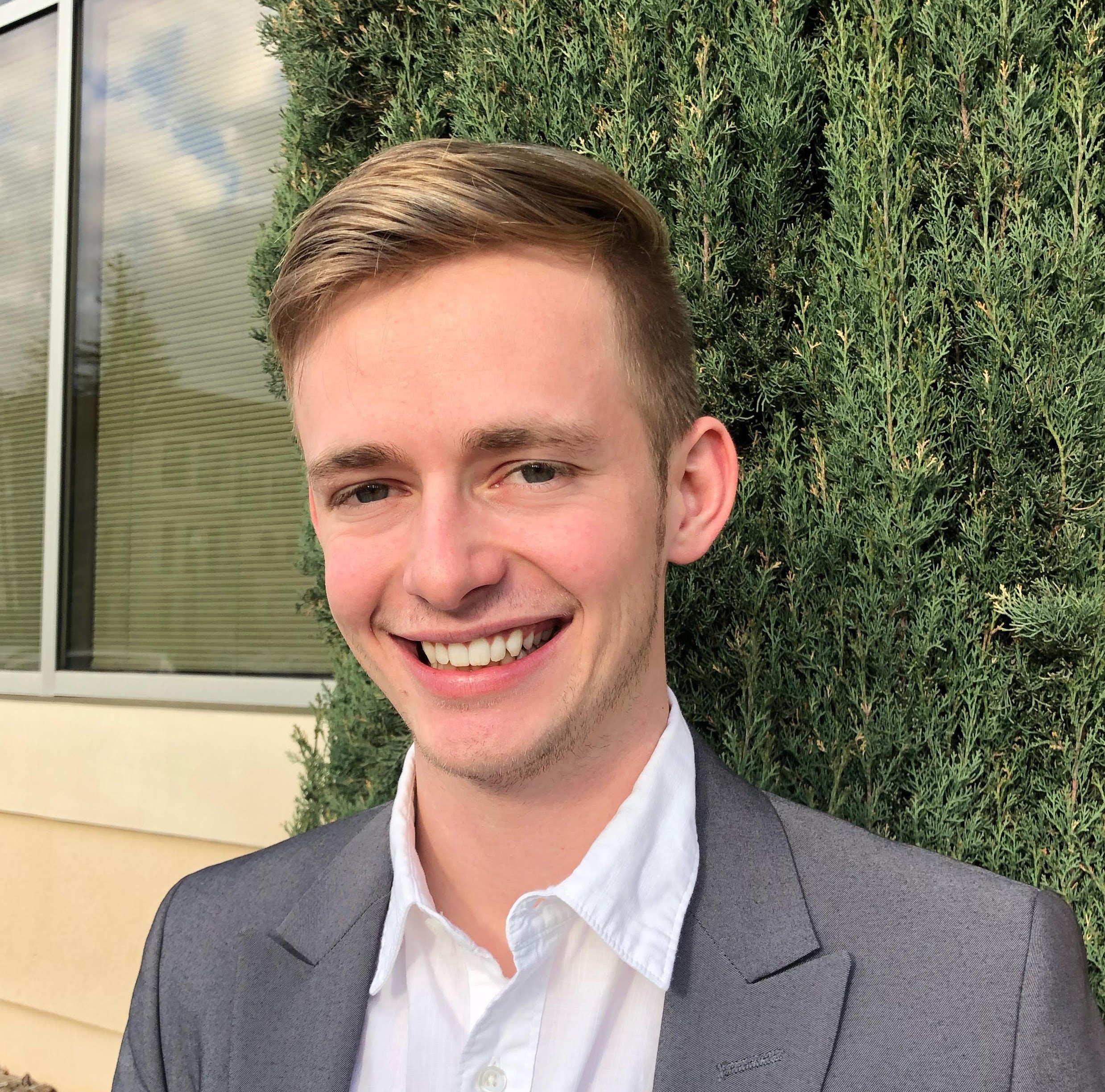
Insights from a series of interviews
Jeff Kampfe is a senior at Santa Clara University. In 2018-2019, as a Hackworth Fellow at the Markkula Center for Applied Ethics, he interviewed six experts on the subject of data ethics. The following is his reflection on part of that project.
Today, data scientists are able to generate some of the most remarkable insights we have ever seen. From creating healthcare solutions to helping solve facial recognition bias, the impact of data scientists can stretch to almost every aspect of our lives. And as with any occupation, there are certain criteria that employers are looking for in a data scientist. A quick LinkedIn search for “data scientist” yielded, among others, the following descriptors:
- “We seek an individual who brings creativity and analytical excellence...”
- “Our ideal candidate is an independent, analytically-minded individual with strong analysis and statistical modeling skills.”
- “The candidate should be confident working alone to solve problems and comfortable presenting their findings to other engineers.”
While creativity, analytical excellence, and problem-solving are all necessary tools for data scientists to possess, there are certain virtues they ought to exhibit as well. By virtues, I mean moral characteristics that are developed through habit and practice rather than through reasoning and instruction. The four virtues most pressing for data scientists to focus on are humility, rigor, honesty, and compassion.
These recommendations are derived from a series of six interviews with experts from various fields that investigated some broad topics about the data ecosystem. The experts interviewed were
- Prof. Shannon Vallor - Technology ethicist and visiting researcher at Google, who specializes in the integration of ethics with industry and engineering/computer science education.
- Mark Nelson - Co-director of the Peace Innovation Lab at Stanford University, whose work focuses on designing, catalyzing, and scaling up collective positive human behavior.
- Jacob Metcalf, PhD - Consultant and scholar specializing in data and technology ethics.
- Prof. Michael Kevane - Scholar of political economy, who also teaches courses on the Economics of Gender in Developing Countries, African Economic Development, and Econometrics.
- Iman Saleh - Research scientist at Intel, who is currently focusing on privacy and ethics for AI.
- DJ Patil - Previous U.S. Chief Data Scientist, who served as Chief Security Officer and Head of Analytics and Data Product Teams at LinkedIn, where he co-coined the term “Data Scientist.”
The goal of these interviews was to see what individuals with unique and insightful viewpoints can teach us about the field data ethics, where it is heading, and what challenges we may face along the way. The virtues of a good data scientist were one of the topics discussed in the interviews. The respondents highlighted four key virtues:
Humility
It’s no secret that data scientists are able to manipulate powerful datasets that can affect everything from our consuming habits to our voting patterns. So as the famous Spider-Man quote goes, “With great power comes great responsibility.” The virtuous data scientist should strive to set pride and arrogance aside in their work. A good data scientist understands the boundaries of their occupation and does not attempt to extend beyond them.
In her interview, Prof. Shannon Vallor stressed the importance of this virtue:
The first would be the virtue of humility. Making sure that as a data scientist, you're not simply focused on what you can predict, what you can control, what you can measure, and what you can analyze. You need to know what you can’t measure, what you can’t control. There must be an understanding of the limits of the tools you're working with and an understanding of the ways in which tools do not always deliver the insights that we hope they will.
Understanding one’s limits encompasses one aspect of humility, but humility also includes not being overly ambitious. Vallor continued:
A good data scientist must understand the ways in which the instruments and the algorithms can behave unpredictably, the way in which they may have effects that we didn't intend, and simply the humility to understand that we as researchers are not gods.
Rigor
The type of rigor important for data scientists has to do with the precision, thoroughness, consistency, and exactness of the methodology practiced within the field. Part of Mark Nelson’s interview helps explain this notion of rigor. When asked about the virtues of a good data scientist, Nelson noted:
The first structural solution is that you make things more ethical by making them more specific and concrete. There is something profoundly ethical about just doing things in the right order and helping people see things in the right order. These are all aspects of ethics that I never hear discussed. The assumption is that everyone has to do the right thing, but the problem is that we assume everyone already knows what the right thing to do actually is.
Nelson’s analysis makes a subtle point in the field of ethics. Often ethics focuses on determining what the ethical course of action is. However, part of practicing ethics is making sure that ethical actions are followed through. Nurturing the virtue of rigor helps ensure that missteps do not occur and proper procedure is followed.
Another aspect of rigor is having the proper skills to complete a task. In his comments on data ethics, Jacob Metcalf highlighted this aspect:
Knowing that even seemingly small design decisions can profoundly affect how people live their lives. That’s not just a matter of principle, it’s a matter of skills. Someone might agree fully with my statement that even small design decisions and algorithmic systems can have significant effects on individuals’ lives and on society, but the ability to see that trend then make a choice is not itself a principle but a practice.
Honesty
The field of data science promotes output. Employees are paid based on what insights they can deliver and academics are compared to their peers within academic circles. With such emphasis on driving results, it is easy to see how dishonest practices might be incentivized. Data scientists stopping at nothing to deliver the insights they desire is where abuses occur. Given this charged atmosphere, Prof. Michael Kevane pointed to the importance of honesty:
... when [data scientists] are surprised by their conclusion, it seems as if something is not “right.” Their work will get changed until they arrive at the answer they initially expected. . . However, the scientific community can’t know everything and they usually presume that data scientists have done all of their robustness checks. The subtle problem is knowing when enough is enough. Being honest to oneself as a data scientist and the scientific community at large is one of the virtues I think a data scientist should have.
Compassion
There are more methods of collecting data than ever before in human history., yet somehow our minds create a gap between data and people. Much of the data created and collected is about people. By dealing with human data, data scientists are really just people scientists. For us to be respected as autonomous human agents, our data needs to be processed in ways that value our autonomy and humanity. As Prof. Vallor explained,
Data represents observations and indications of human life and activity. It reveals things about individuals who have moral status, dignity, and the right to be treated as such. I think good data scientists never forget that. They never forget the people behind the data and the moral respect that they are owed.
Good data scientists consider the social implications of their work. Compassion requires humans to act in the interest of other humans. To create a system of compassion within data science, abuse prevention, and consumer protection must be continuously considered throughout the data pipeline. Iman Saleh discussed this in her interview:
we [engineers] know now how to check for some biases. There are still a lot of human factors and biases that are dependent on the application that may require further discussion with social scientists, anthropologists, and ethicists that companies are starting to hire. But in general, there are some best practices; for example, if you are collecting data and you know where your application is going to be deployed in the US for example, you ask yourself “Does this data really reflect the demography of the US?”
True compassion lies in acknowledging all humans. In his interview, DJ Patil emphasized this notion of acknowledgment and respect:
We have to be very careful because when we are building something, it is easy to say “Eh, that’s just an edge case.” But the edge cases have names. The edge cases have families. We cannot lose sight of that.
A resume will usually tell us whether someone has the skills to be a data scientist. Those skills could come from work experience, classes, or perhaps a few YouTube videos. However, virtues are equally important to being a good data scientist--yet much harder to cultivate. Being virtuous requires a deliberate mindset and practice. As our data collection systems and analytical tools grow more advanced, key virtues must be valued in conjunction with hard skills.