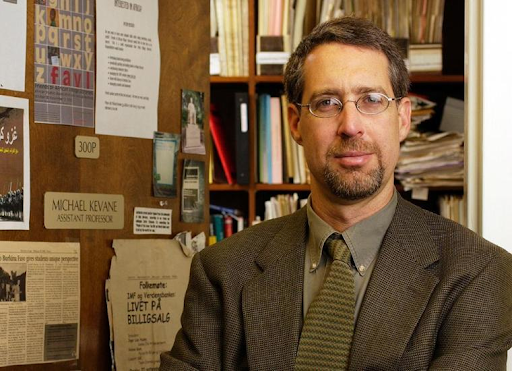
"These issues are complicated, and we have to acknowledge that there are tradeoffs."
Welcome to "Cookies, Coffee, and Data Ethics"! My name is Jeff Kampfe and I am a senior at Santa Clara University, studying Economics and Philosophy. I am also a Hackworth Fellow at the Markkula Center for Applied Ethics. This article is the third in a series of interviews that involve hot coffee, tasty baked goods, and the complex issue of data ethics. The goal of these interviews is to see what individuals with unique and insightful viewpoints can teach us about the field data ethics, where it is heading, and what challenges we may face along the way. Thanks for stopping by!
The following is an edited transcript of a conversation with Professor Michael Kevane.
At Santa Clara University, Michael Kevane teaches courses on the Economics of Gender in Developing Countries, African Economic Development, and Econometrics. He has published articles on the performance of rural institutions and markets, and is the co-author of books such as Women and Development in Africa: How Gender Works and Rural Community Libraries in Africa (with Valeda Dent and Geoff Goodman). He also writes and gives talks on various aspects of the political economy of Sudan and Burkina Faso. His current studies focus on ways in which access to books, libraries, and reading programs affect student performance. He is the founder and director of the non-profit organization Friends of African Village Libraries.
Can you tell me a bit about your past experience with data science?
As a PhD student back in the 1980s and 90s training in economics, I was part of the first generation of students that didn’t have to use punch cards to do statistical analysis. It was the beginning of statistics software, statistical computing software packages, the first spreadsheets, Lotus 123, and by the late 80s most PhD students could do computer driven statistical analysis. Before that it was pretty hard because you had to use a mainframe and it was often time consuming and expensive. I feel as if I have been a beneficiary of that revolution that has continued rapidly, especially seeing how the computing power available to people now is hundreds of times greater than what was available to me 20 years ago. In addition to that processing power, the internet has also meant that there has been a great increase in data available for processing. That has lead us to this world now of Big Data where every company that operates on the internet is generating, harnessing, or collecting data on a minute by minute and second by second basis.
The amount of data that has been created requires new tools for big data analysis. These tools run the gamut from using millions of Facebook or Twitter posts to come up with scores for aggregate mood trends all the way to predictive-type analysis such as using Google searches to determine where flu hot spots are. The first thing people do these day when they get sick is Google their symptoms, so if you can track those kinds of searches you can get a jump on where infectious diseases might be more likely. Overall I would say data practices have been through a huge revolution and it’s exciting to have lived through it.
How has that background, of essentially growing up with the data revolution, influenced you work in Burkina Faso?
Well the first kind of quantitative research that I did was back in Eastern Sudan in 1985. I performed a large survey on several hundred farmers and I had to do everything by hand. I interviewed farmers with a paper questionnaire, transferred all the data by hand to graph paper and made a spreadsheet, and finally calculated all of the aggregates, standard deviations, and statistical analysis via a handheld calculator.
I have moved from that to my most recent project which involves a web scraping of the registry of about 6 million voters within Burkina Faso. The Burkina Faso registry is publicly available and so we used that database to determine people’s ethnicities based on their last names. It turns out in Burkina Faso that one’s name is also a good indicator of one’s ethnicity. The issue of how ethnicity matters for outcomes like voting or the distribution of government public goods has been a long standing concern for public scientists and economists. This is because public goods are important for development outcomes, such whether one’s villages gets a clinic or a school.
So knowing how public goods and voting outcomes align with the nearly 60 ethnic groups within Burkina is very important. With this large database of 6 million names we can write algorithms that assign some people to ethnicities based on well known ethnographic understanding of what names correlate to which ethnicities. Even when we don’t know what ethnicity some names are linked with, we can use algorithms to determine if a name is commonly associated with names of people whose ethnic groups we do know. If these two names are always seen in the same villages, then it is a reasonable supposition to say that the name we didn’t know probably belongs to that same ethnic group of the name we did know. Now, you may think “well why not just ask people?” but we are finding that there are around 20 thousand names that are not assigned. If you were to conduct and interview and it takes an hour to find people with a given name, then 20 thousand hours ends up looking like a long time. Using an algorithm to assign ethnicities on the basis of the data itself is a very efficient substitute for this process.
What do you think are some of the virtues or values that make a good data scientist? What do those look like in practice?
The first virtue that I can think of is not lacking a sense of ethics. The rewards for coming up with certain conclusion in data analysis are very high. If you are the first person to come up with analysis that is found to be groundbreaking, perhaps that a given illness is far more contagious for a certain ethnic group, you might get a lot of notoriety and press. So the temptations to make up or change data are very strong. The risk today of being caught changing numbers in order to create a strong conclusion or result also isn’t very high. Many times the motivation for changing data stems from good intentions and a simple attempt to prove one’s theory. But you obviously don’t want people who are doing data analysis to engage in this completely unethical behavior of changing data.
There is also a second, more subtle unethical behavior. One can fall into what is known as positive confirmation bias where a researcher has an idea about a certain type of relationship and keeps doing data analysis and research until they arrive at the conclusion they wanted to arrive at. The variables get changed and datasets get rearranged until the result the researcher set out to find is arrived at. This happens more often than you would think. Data analysts will often look for the answer that they expect to find. Then, if they are surprised by their conclusion, it seems as if something is not “right.” Their work will get changed until they arrive at the answer they initially expected. Another problem with this is that we rely on the scientific community to point out the flaws in practices such as these. However, the scientific community can’t know everything and usually presumes that data scientists have done all of their robustness checks. The subtle problem is knowing when enough is enough. Being honest with oneself as a data scientist and with the scientific community at large is one of the virtues I think a data scientist should have.
What are your thoughts on data ownership? What are some of the largest issues that need to be resolved surrounding this topic?
I think these are very important and interesting issues. However, sometimes they can get overblown. I can imagine nefarious scenarios that are not quite as nefarious as we might imagine. It is sometimes appropriate to note that we don’t have to shut down all data analysis because someone’s privacy might be invaded. One of the reasons why I push back against something like this has to do with something I encountered while running a study-abroad program in Burkina Faso. The program combined economics and photography, so one of the side benefits for me was to learn from a professional photographer. One of the things I learned early on was that professional photographers had to fight for decades for the right to take photographs of people when they are in public.
This is not a right around the world, but in the United States if you are in public a person has the right to take your photograph. It is important to remember why we have that right. On one hand we want privacy, on the other hand we want sunshine. Things like the Black Lives matter movement are aided by the fact that people who are being abused in public can be filmed and the information can be spread. We would think it wouldn’t be a good idea if these abusers could claim that their actions couldn’t be filmed even though they were doing them in public. I think this also translates to self driving cars. The whole premise of the self driving car is having a machine continuously take images of the public space. Do those images of what people are doing in public spaces somehow belong to the individual whose image was taken? There is a tension with privacy that is inevitable, and striking a balance is important. These issues are complicated and we have to acknowledge that there are tradeoffs.
How might we as a society balance the benefits that data analytics gives us (in health care, security, efficiency, innovation) against some of the potential threats it poses to privacy and autonomy?
I would say there is no global answer that applies to all sectors. Off the top of my head I could think of a few sectors where helpful steps could easily be taken. One of these might be medical data. On the one hand, we want data analytics people to do large scale analytics on medical information because that’s how we learn a lot about diseases. By tying medical treatments to genetic information and other information we have about people, we can improve our already stupendous accomplishments in bettering health and increasing life spans. Of course, the downside of that is that if someone is to have a genetic condition that is very highly associated with cancer they might want their data to be more private. We as a society are having to struggle with eliminating genetic information and preexisting conditions in access to health care. I think we moved in this direction with the Affordable Care Act, where insurance companies can no longer base their decisions on preexisting conditions. Moving towards a universal health care system, whether it be by private insurance, people being placed in government insurance pool, or a combination of the two, helps everyone be guaranteed a certain level of peace of mind. One’s access to healthcare shouldn’t be determined by genetic information or preexisting conditions, and that helps eliminate some of the privacy concerns.
Another issue we are having is the issue of Net Neutrality. Traffic on the internet can’t be determined by willingness to pay and companies shouldn’t use predictive analytics to make individualized rules for the internet. The rules for the internet should be independent of the participants of the internet. If you look back in history, the United States decided that electricity was important enough that it would be provided to everyone. The same goes with the postal service. No matter where you lived in the United States, you were going to have access to this essential medium of communication. There are plenty of examples where we have had to balance the individualized market-driven solution versus the open accesses-solution, and I think those are important concepts to take into consideration in this area.
Is it ethical for organizations (incl. companies, governments, nonprofits, etc.) to keep data sets indefinitely? If not, how should that issue be addressed?
Well first off this isn’t really my area of expertise. However, part of me is attracted to the idea that electronic data should have a shelf life. This would make it so that data has a cost associated with its preservation. Data storage isn’t simply electronic, however. Every family most likely has a set of records of some sort that denote family members. This would seem to be a sort of long term data preservation. So I don’t think anyone is saying that all data has to be erased after three years or something similar. But I like the idea of moving away from our current situation where electronic data is virtually costless to store. The advances in electronic storage have been so rapid that some companies’ business model is simply to provide free storage forever in exchange for using their service. So at some point we might want to say that electronic data has something near a five year limit and then it needs to be erased.
For prior articles in this series, see "On Data Ethics: An Interview with Jacob Metcalf," and "On Data Ethics: An Interview with Shannon Vallor."