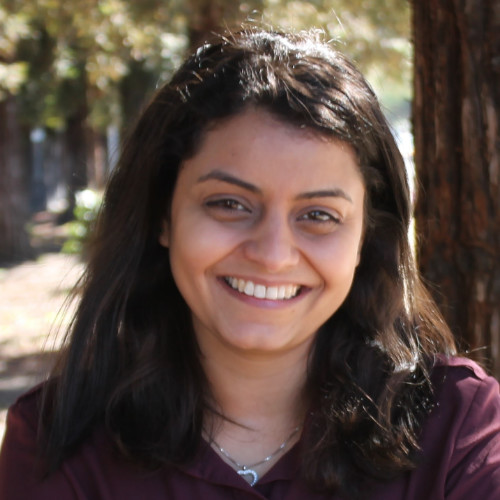
“Automatic deletion might be scary, but I think it’s the right thing to do.”
Welcome to "Cookies, Coffee, and Data Ethics"! My name is Jeff Kampfe and I am a senior at Santa Clara University, studying Economics and Philosophy. I am also a Hackworth Fellow at the Markkula Center for Applied Ethics. This article is the fifth in a series of interviews that involve hot coffee, tasty baked goods, and the complex issue of data ethics. The goal of these interviews is to see what individuals with unique and insightful viewpoints can teach us about the field data ethics, where it is heading, and what challenges we may face along the way. Thanks for stopping by!
The following is an edited transcript of a conversation with Iman Saleh.
Iman Saleh is a research scientist at Intel, focusing on privacy and ethics for artificial intelligence. She holds a PhD in software engineering, has authored more than thirty technical publications, and has many years of experience designing and building data-centric solutions. Iman is recognized by the International Association of Privacy Professionals as a Fellow of Information Privacy and has always been passionate about developing algorithmic solutions for preserving privacy when building software systems.
Can you tell me a bit about your experience with data science?
My background is software engineering, and I work with computer vision applications that use machine learning. I did some work on privacy protection and data mining while earning my Master’s degree and this is where I was exposed to the side effects of technology. So, besides just solving technical problems, I learned about understanding the social impact of these technical problems and how to find algorithmic solutions to address it. I was given the opportunity to work on research questions related to how autonomous driving affects privacy. The project consisted of autonomous cars driving along the roads taking pictures of people, other people’s license plates, and other private information. My task was figuring out how to handle and process that data in a way that was sensitive to people’s privacy.
Based on my work, I started building privacy-preserving tools for the computer vision capabilities. Then, since I was working on that, I was recruited to join a cross company effort on Ethical AI. The effort was led by a team of people from different departments, backgrounds, and interests who are passionate about studying and addressing the ethical implications of technology. There's a lot of research around AI now, since it often deals with personal information and companies are concerned with the implications of that. They look at how to solve this issue either through design, legal, or algorithmic methods. I joined that team to focus on the algorithmic solutions. I work with a team of policy makers, legal people, anthropologists, and social scientists, and I represent the engineering side of things. I have continued to work on that and expanded beyond privacy into issues like bias, explainability of models, and transparency that needs to be built into algorithms.
Is it possible to develop a set of practices around what makes a good data scientist? If so, what do those look like? What are the virtues of a good data scientist?
At this point, I don't think we can assume that people have perfect sensitivity to ethical issues. Everyone wants to do the right thing, but they might not have the knowledge to enable them to do the right thing. This includes engineers and data scientists. I like to make the analogy to privacy and security. They are ethical issues: if you are collecting data from your customer, you have to make sure to protect it. You need to make sure you have consent. It needs to be used in the right way; it is not to be shared with a third party without consent. While this may now have a legal aspect to it, it started with being ethical and doing the right thing.
We can draw lessons from these areas because they are well established. The process started with teams who may not have had the security and privacy education, so they usually looked toward a centralized entity that went through projects and reviewed the security and privacy. But then over time people became more informed, and now there are security architects and privacy champions in every company. Some of them can be embedded in teams and they become the voices pushing for and teaching the team about the privacy concerns, what the team needs to look for, when to raise a flag, and so forth.
I think ethics will go the same route. Now, because there is no consensus, it is done in a very centralized way. Every company has their own ethical committee or privacy office where if you are building a product that has some sort of social implications, you go through that office for review. But I think over time some of these practices can be built into the process itself. For example, let's look at checking for bias and transparency. It is getting more and more mature; we [engineers] know now how to check for some biases. There are still a lot of human factors and biases that are dependent on the application that may require further discussion with social scientists, anthropologists, and ethicists that companies are starting to hire. But in general, there are some best practices; for example, if you are collecting data and you know where your application is going to be deployed in the US for example, you ask yourself “Does this data reflect the demography of the US?” And there is also being sensitive to what kind of training you do for your models, depending on where you are going to deploy said models.
I think eventually ethics will be embedded in the software development life cycle. Starting from the social scientist view of what should be done or what policy we need to apply as a company or as an organization, we should take that and implement it in the product life cycle at each phase. Either it gets addressed by putting in place contracts and processes, or it can be addressed algorithmically or by features and new use cases that could be implemented just to address these ethical concerns.
What are your thoughts on data ownership? What are some of the largest issues that need to be resolved surrounding this topic?
Data ownership is definitely an unresolvable issue. Data about you isn’t necessarily owned by you. It is a problem, because even if we want to solve it and say “If I put anything about me on the web and I own it, I have copyright over it, I have control over how it is used,” there is no logistically feasible way to enforce this. My take on that so far is that companies will be incentivized to act responsibly when dealing with private data to preserve their customer base. So far the regulation is not strong enough to protect us as individuals. GDPR is trying to push in the direction of emphasizing consent, but it is still not universal. The motivation for companies to do the right thing still comes largely from their bottom line and protecting their business.
Is it ethical for organizations (incl. companies, governments, nonprofits, etc.) to keep data sets indefinitely? If not, how should that issue be addressed?
Data purging strategy needs to be specified early on, when you collect the data. The more data you have, the more liability you have. Companies are becoming aware of this and that now it may not be a good thing to keep all the data. There's also a saying that data ages like milk. Old data may not be as useful and applicable as distributions change over time.
At the same time, engineers have a hard time deleting data. It becomes then a balance between liability and the engineering requirements where application development processes are always hungry for more data.
If you make the case that all data is not that useful anyways and the risk of keeping data outweighs the benefits, then it should be deleted. Newer systems come with a data purging options, and many companies that create new data documents now ask how long the document will live for and the document is deleted automatically according to this metadata. Automatic deletion might be scary, but I think it’s the right thing to do.
How might we as a society balance the benefits that data analytics gives us (in health care, security, efficiency, innovation) against some of the potential threats it poses to privacy and autonomy?
I think this concern is not new. When computers started infiltrating our lives, people thought that the technology would take over the world. I think some of these fears are overblown, and I don’t see AI taking over our world anytime soon. AI is still very specialized. You have one model that does one thing very well, and it is far behind compared to the human mind. I do have concerns about the effect on jobs, and the introduction of bias--you now have automatic processes deciding whether you get a loan or not, or get enrolled in a school or not--and taking the human factor out of these life decisions is risky. To be honest, I think we will come to a place of balance. For decisions that have big social consequences, you will find a human being needed in the loop. If, say, you can detect that you are always rejecting women in job applications, these flags will trigger some sort of human response. As for jobs, what smart countries should do is redirect their workforce. For example, we still need people to build huge amounts of labeled data for AI models to work. Labeling data is a new job that has been created just because AI exists. Even though some jobs will disappear, new jobs will also be created. The smartest thing is to provide learning programs, to change our curriculum in schools, and to make people ready for the jobs of the future.
Does describing people as data points lose a sense of their humanity/dignity? Or is that simply just part of the process when doing data science?
Data has been considered for so long as just a component for the development process. You have the data, that goes into a pipeline, and it gets presented in a certain way. With AI, now more than ever, data relates to humans. Huge amounts of data relate to human life, human behavior. So that’s what we are trying to fix with training: We are trying to train data scientists and engineers to look at data as not as numbers and digits and binary, but as a human artifact. When you are building these processes, you are affecting human lives. And that is a huge shift in mentality, and that is what we have to deal with.
We are all biased. We all have a limited view of the world based on who we are and what we understand. So the best technique to address these biases is actually not a technical solution but a social one; these systems need to be developed by a diverse team. That’s the best way to overcome any unwanted bias, consequences, or scenarios we may not have thought of. It’s the collective sensitivity of the team that ensures we build good models that are applicable for the majority of people. In other words, the development team has to reflect the society where an application is to be deployed.
What specific issue within data science are you currently interested in?
I think some of the challenges we have come from the disconnect between social scientists and ethicists on one side, and engineers and data scientists on the other side. We have two different voices that are speaking two different languages. One of the areas that needs to be improved is bridging the gap between these two groups. There are so many great ideas from the ethicists’ side, and sometimes they provide the answers, but they don’t know how to translate that into technical solutions. On the other hand, engineers can do the right thing and have the tools to implement creative solutions, but sometimes they don’t necessarily know what that right thing is. That's one area where I am focusing right now, translating between these two groups of experts.
I am also interested in transparency and explainability of deep learning models. There is a lot to be done on that front. There is a lot to be done with certification, too. Also, we need to deal with context, and determine the applicability of deep learning models to specific domains. If one model is used to help cars autonomously navigate the streets of the US, for example, that doesn’t mean it will carry over to the EU’s streets. Context modeling is one area that needs more work.
For prior articles in this series, see "On Data Ethics: An Interview with Jacob Metcalf," "On Data Ethics: An Interview with Shannon Vallor," "On Data Ethics: An Interview with Michael Kevane," and “On Data Ethics: An Interview with D.J. Patil.”